Gridded crop modelling to simulate impacts of climate change and adaptation benefits in ACASA
Gridded crop modelling builds an understanding of how climate change impacts crops, helping researchers to adapt agricultural methods and combat food insecurity.
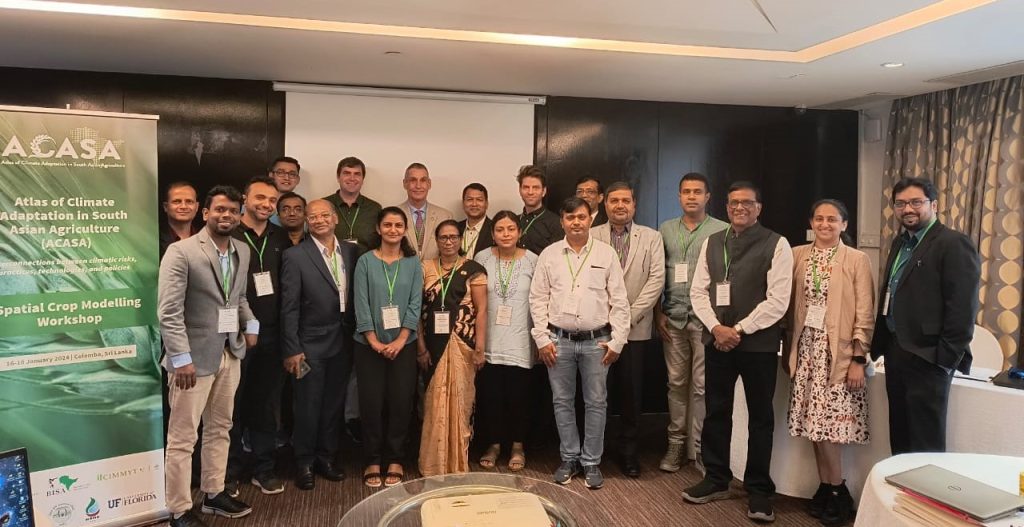
Global temperatures are projected to warm by between 1.5-2 degrees Celsius by the year 2050, and 2-4 degrees Celsius by 2100. This is likely to change precipitation patterns, which will impact crop yields, water availability, food security, and agricultural resilience.
To prepare for these challenges, the Atlas of Climate Adaptation in South Asian Agriculture (ACASA) project use process-based simulation models that can predict crop growth, development, and yield in order to understand the response of crops to climate change. Models such as Decision Support System for Agrotechnology Transfer (DSSAT), InfoCrop, and Agricultural Production Systems Simulator (APSIM) facilitate the field scale study of the biophysical and biochemical processes of crops under various environmental conditions, revealing how they are affected by changing weather patterns.
The ACASA team, along with experts from Columbia University and the University of Florida, met for a three-day workshop in January 2024 to boost the work on spatial crop modelling. The aim was to design modelling protocol through a hands-on demonstration on high-performance computers. . When scientifically executed, gridded spatial crop modelling – even though complex and data-intensive – can be a great way to frame adaptation and mitigation strategies for improving food security, which is one of the ACASA project’s goals.
Decisions on data
The group decided to use DSSAT, APSIM, and InfoCrop for simulating the impact of climatic risks on crops such as rice, wheat, maize, sorghum millet, pigeon pea, chickpea, groundnut, soybean, mustard, potato, cotton, and more. They chose harmonized protocols across all three models with standard inputs, such as conducting simulations at 0.05 degrees. The model input data about weather, soil, crop varietal coefficients and crop management are being collected and processed for model input formats at 5 kilometer (km) spatial resolution.
A Python version called DSSAT-Pythia is now available to accelerate spatial and gridded applications. The programming for implementing InfoCrop on the Pythia platform is in progress. InfoCrop has been proven in India for past yield estimations, climate change spatial impact, and adaptation assessments for 12 crops.
For other crucial modelling components, a work plan was created including developing regional crop masks; crop zones based on mega-commodity environments as defined by CGIAR; production systems; crop calendars; and irrigated areas by crop. Genetic coefficients will then be calculated from measured past values and recent benchmark data of varietal units.
With this information, several adaptation options will be simulated, including changes in planting dates, stress-tolerant varieties, irrigation, and nitrogen fertilizer (quantity, methods, and technology), residue/mulching, and conservation tillage. The team will evaluate impact and adaptation benefits on yields, water, and nitrogen use efficiency based on the reported percentage change from the baseline data.
As the project progresses, this work will make strides towards realizing food security for the planet and increasing the resilience of smallholder farming practices.
Authors: Anooja Thomas, University of Florida; Apurbo K Chaki, BARI, Bangladesh; Gerrit Hoogenboom, University of Florida; S Naresh Kumar, ICAR-IARI, India